What is black box insurance? It’s a novel approach to risk assessment and insurance, leveraging data analysis to redefine how we approach coverage. Imagine a system where your driving habits, not just your location, determine your auto insurance rates. This innovative model is built on the collection and analysis of vast amounts of data to predict and manage risks more precisely.
While seemingly opaque, it promises tailored policies and potentially lower premiums for responsible behavior.
This exploration dives into the intricacies of black box insurance, outlining its core principles, benefits, drawbacks, and future implications. We’ll examine how data collection and analysis shape insurance premiums and coverage, shedding light on both its potential advantages and the challenges it presents.
Defining Black Box Insurance
Black box insurance operates on a fundamentally different principle compared to traditional insurance models. It relies on data collection and analysis from various sources to assess risk and adjust premiums. This approach differs from traditional methods, which often rely on factors like driving history and vehicle characteristics. This departure opens new possibilities for risk assessment, but also raises crucial questions about data privacy and fairness.The core principles of black box insurance models revolve around the extensive use of telematics data, including driving patterns, location, and vehicle usage.
This data is then analyzed to create personalized risk profiles for individual policyholders. The aim is to tailor premiums to the actual risk each driver presents, rather than relying on generalized assumptions. However, this necessitates a sophisticated approach to data handling, ensuring accuracy, security, and equitable application.
Black Box Insurance Definition
Black box insurance is an insurance model that assesses risk based on data collected from various sources, without explicitly disclosing the specific factors used in the risk calculation. This opaque nature distinguishes it from white box insurance, which clearly defines the risk factors and their corresponding weightings. The key is to use sophisticated algorithms and data analysis to determine risk, without necessarily making the underlying rationale transparent to the policyholder.
Core Principles of Black Box Models, What is black box insurance
The core principles of black box insurance models rely heavily on the collection, processing, and analysis of vast amounts of data. This data often includes GPS location data, driving behavior, and other information collected from vehicles, mobile devices, and wearables. The model then uses complex algorithms to analyze this data and assign risk scores to individual drivers. These scores are crucial for determining insurance premiums.
The specific algorithms remain largely hidden from the policyholder, hence the “black box” moniker. This approach aims to create a more personalized and accurate assessment of risk. Examples include evaluating the driver’s acceleration, braking, and cornering habits. The data points are combined to generate a comprehensive risk profile, allowing for dynamic adjustment of insurance premiums.
Key Characteristics of Black Box Insurance
Black box insurance is characterized by its opacity and reliance on complex algorithms. The methods used to assess risk and set premiums are not readily apparent to the policyholder. This characteristic contrasts sharply with white box insurance, where the factors influencing premium calculation are explicitly Artikeld. A key feature is the use of advanced data analytics to uncover patterns in driving behavior and correlate them with risk.
So, like, black box insurance is this thing where they kinda just… track your driving habits, right? It’s all about using data to figure out if you’re a good driver or not. Lowkey, it’s kinda related to how much you rock that China Glaze neon nail polish, china glaze neon nail polish. If you’re extra careful, you might get a lower rate.
But if you’re like, always zooming around, then it’s gonna be a higher premium. It’s all about that data game, ya know?
This can lead to more accurate and personalized insurance premiums. The algorithms themselves are often proprietary, safeguarding the competitive advantage of the insurance company. Another distinguishing characteristic is the continuous refinement of the models based on ongoing data collection and analysis.
Comparison with White Box Insurance
White box insurance, in contrast, operates with a clear and readily accessible explanation of the factors determining premiums. This transparency allows policyholders to understand the rationale behind their premiums. In contrast, black box insurance uses a more opaque approach. The premiums are based on a more nuanced analysis of data, often through machine learning algorithms.
Characteristic | Black Box Insurance | White Box Insurance | Examples | |
---|---|---|---|---|
Risk Assessment | Based on complex algorithms and data analysis; opaque to the policyholder | Based on explicit risk factors and their weights; transparent to the policyholder | Driving behavior, vehicle usage, location data | Age, driving history, vehicle type |
Premium Calculation | Dynamic and personalized based on continuously updated risk profiles | Static and based on pre-defined factors | Dynamic adjustment based on real-time data | Fixed premiums based on age and driving history |
Transparency | Low; the internal workings of the model are not disclosed | High; the factors determining the premium are clearly defined | Policyholders do not know the specific data points influencing their premium | Policyholders know exactly how their age and driving history affect their premium |
Examples | Telematics-based insurance, Usage-based insurance | Traditional insurance policies, policies based on driving history | Insurance companies using GPS and sensor data | Insurance companies using driving records from DMV |
Coverage and Exclusions
Black box insurance policies, centered around telematics data, offer a unique approach to assessing risk and pricing premiums. Understanding the specific coverage types and exclusions is crucial for policyholders to make informed decisions. This section delves into the details of common coverage components, highlighting the nuances of these policies and outlining potential limitations.Coverage under black box insurance policies is typically tailored to address the specific risks associated with vehicle use.
Policy terms and conditions, including coverage details and exclusions, are crucial for understanding the extent of protection. Factors such as driving behavior, location, and vehicle type significantly influence the premiums and coverage amounts.
Common Coverage Types
Black box insurance policies often include coverage similar to traditional insurance, but with a strong emphasis on safe driving habits. Common types include liability coverage, comprehensive coverage, and collision coverage. Liability coverage protects against financial responsibility for damages caused to others. Comprehensive coverage addresses non-collision damage, such as vandalism or theft. Collision coverage protects against damage to the insured vehicle in an accident.
The specific scope of each type can vary across policies.
Typical Exclusions
Black box insurance policies may exclude certain types of events or situations. Common exclusions include intentional acts of vandalism or damage, damage resulting from off-road use, use of the vehicle for illegal activities, and pre-existing conditions of the vehicle. Exclusions often specify circumstances where the insurance coverage will not apply. It is vital to carefully review the policy document to understand these limitations.
Factors Influencing Coverage Amounts and Premiums
Several factors contribute to the determination of coverage amounts and premiums in black box insurance. These include the driver’s risk profile, vehicle type, location, and the specific driving behavior tracked by the black box device. A driver with a consistently safe driving record will often receive lower premiums. Similarly, a newer, more expensive vehicle may result in higher coverage amounts.
Sample Black Box Insurance Policy
Coverage | Description | Exclusions | Notes |
---|---|---|---|
Liability | Covers financial responsibility for damages caused to others. | Intentional acts of damage, use for illegal activities. | Limits may apply based on severity of accident. |
Comprehensive | Covers non-collision damage to the vehicle. | Pre-existing damage, damage due to off-road use. | Deductibles and coverage limits may apply. |
Collision | Covers damage to the insured vehicle in an accident. | Damage caused by intentional acts, damage resulting from racing. | Deductibles and coverage limits apply. |
Scenarios Where Black Box Insurance Might Not Apply
- Use of the vehicle for illegal activities, such as racing or transporting contraband.
- Intentional acts of damage or vandalism to the vehicle.
- Damage resulting from use of the vehicle in unauthorized or inappropriate ways (e.g., off-road driving when not permitted by the policy).
- Pre-existing conditions or damage to the vehicle prior to the policy’s effective date.
- Damage caused by a covered peril, but where the driver acted recklessly or negligently, exceeding the permitted limits.
Data Collection and Analysis: What Is Black Box Insurance
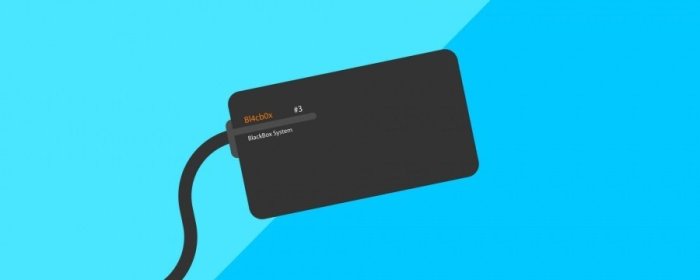
Black box insurance relies heavily on the meticulous collection and sophisticated analysis of data to assess risk and accurately calculate premiums. This data-driven approach allows insurers to personalize premiums based on individual driving behaviors, rather than relying solely on demographics. The accuracy and fairness of these assessments are paramount to the success and public perception of the insurance model.The process of collecting and analyzing data is complex and multifaceted, demanding a rigorous approach to ensure accuracy, fairness, and security.
This involves various methods, sophisticated algorithms, and adherence to strict privacy regulations. Understanding the nuances of this process is crucial for comprehending the functioning of black box insurance.
Data Collection Methods
Data collection for black box insurance typically involves a combination of technologies and methods. The most common method is the utilization of telematics devices installed in vehicles. These devices continuously monitor various driving parameters, including speed, acceleration, braking, and location. Data collected through these devices is transmitted wirelessly to the insurance provider’s servers. This real-time data collection provides a comprehensive picture of a driver’s driving habits, allowing for a more accurate assessment of their risk profile.
Data Analysis Algorithms
Sophisticated algorithms are employed to analyze the massive amounts of data collected from telematics devices. These algorithms are designed to identify patterns and trends in driving behavior, which can be used to categorize drivers into risk profiles. For example, drivers exhibiting frequent speeding or aggressive driving styles might be classified as higher-risk drivers. Statistical models and machine learning techniques are frequently utilized in this process, allowing for the identification of complex relationships between driving behavior and accident risk.
Data Security and Privacy
Data security and privacy are paramount in black box insurance. Insurance companies must adhere to stringent regulations and implement robust security measures to protect sensitive driver information. This includes encrypting data transmissions, employing secure storage systems, and adhering to privacy policies that comply with industry standards and legal requirements. Protecting customer data from unauthorized access and breaches is critical to maintaining trust and reputation.
Breaches can have significant financial and reputational consequences.
Impact on Premium Calculations
Data collection directly impacts premium calculations. The analysis of driving behavior data allows insurers to tailor premiums to individual risk profiles. Drivers with safer driving habits, as indicated by the data, may qualify for lower premiums, while drivers with higher-risk behaviors may face higher premiums. This personalized approach to premium calculation aims to create a more equitable and accurate system, incentivizing safe driving practices.
Examples of Data Sources
Various data sources contribute to the black box insurance model. In addition to telematics data, insurers might leverage GPS data for route analysis, weather information for road conditions, and even accident data for correlating factors with driving patterns. This multifaceted approach allows for a comprehensive evaluation of driving risk, leading to more accurate premium calculations. Examples of data sources include:
- Telematics Data: Speed, acceleration, braking, location, and time spent driving are key pieces of information collected.
- GPS Data: This data helps identify driving routes, driving patterns, and potentially hazardous driving areas.
- Accident Data: Historical accident data allows for correlation analysis and the identification of potential risk factors.
- Weather Data: Weather conditions, such as rain or snow, can affect driving conditions and impact accident rates.
Benefits and Drawbacks
Black box insurance, characterized by its reliance on telematics data, presents a unique set of advantages and disadvantages compared to traditional insurance models. This approach allows for a more nuanced assessment of risk, potentially leading to more personalized and cost-effective coverage. However, the use of data-driven risk evaluation also raises concerns regarding transparency, accountability, and potential biases. A careful analysis of these benefits and drawbacks is crucial for both policyholders and insurers.
Advantages for Policyholders
The core advantage of black box insurance lies in its potential for customized premiums. By tracking driving behavior, insurers can assess individual risk profiles with greater precision. This allows policyholders who demonstrate safe driving habits, such as maintaining a consistent speed and avoiding harsh braking, to benefit from lower premiums. Furthermore, the availability of detailed driving data can empower policyholders to improve their driving skills and reduce their insurance costs over time.
Real-world examples include drivers who, through consistent safe driving habits, observe a significant reduction in their insurance costs compared to those who demonstrate risky behavior.
Disadvantages and Potential Risks
Despite the advantages, black box insurance presents several potential risks. One significant concern is the potential for bias in data analysis. Factors like demographics, location, or vehicle type could inadvertently influence the assessment of risk, leading to unfair pricing practices. Moreover, the reliance on technology introduces the risk of technical malfunctions or data breaches, potentially jeopardizing the security of sensitive personal information.
Another concern is the lack of transparency in how data is collected, analyzed, and used to determine premiums. This lack of transparency can erode trust and create difficulties in disputing insurance decisions.
Comparison to Traditional Insurance
Traditional insurance models typically rely on factors like age, location, and vehicle type to assess risk. This approach can be less precise than black box insurance, potentially leading to higher premiums for individuals with low risk profiles. However, traditional insurance often provides a wider range of coverage options and benefits, sometimes including more comprehensive accident coverage. A key difference lies in the level of individual control and insight into the factors influencing premiums.
Transparency and Accountability Issues
The lack of transparency in how black box insurance data is used can be problematic. Policyholders may not fully understand the criteria used to determine their premiums, leading to a lack of accountability on the part of insurers. Furthermore, the potential for algorithmic bias in data analysis raises concerns about fairness and equity. Issues of data privacy and security must also be addressed.
Benefits and Drawbacks Comparison Table
Feature | Black Box Insurance | Traditional Insurance | Discussion |
---|---|---|---|
Premium Accuracy | Potentially more accurate due to real-time driving data | Less accurate due to limited data points | Black box offers granular insights into driving behavior for more precise risk assessment, leading to more personalized premiums. |
Cost | Potentially lower premiums for safe drivers | Premiums may be higher for some drivers due to broader risk assessment | Personalized premiums can incentivize safe driving and reduce overall insurance costs for the insured. |
Transparency | Lower transparency regarding premium calculation | Generally higher transparency in pricing factors | Less clarity about how data is used in determining premiums can be a significant concern for policyholders. |
Flexibility | Potentially more flexible coverage options based on usage patterns | Fixed coverage options with limited customization | Data analysis allows insurers to offer customized plans that cater to specific driving habits and needs. |
Applications and Use Cases
Black box insurance, leveraging data analytics and telematics, is rapidly expanding its applicability across various sectors. Its ability to assess risk more accurately and tailor premiums dynamically offers significant advantages over traditional insurance models, particularly in situations where traditional methods struggle to capture the nuances of risk profiles. This adaptability makes it a promising tool for optimizing risk management strategies and enhancing customer value.
Industries Frequently Using Black Box Insurance
Black box insurance’s effectiveness stems from its ability to collect and analyze real-time data on driving behaviors and usage patterns. This granular data allows insurers to assess risk more precisely than traditional methods, often leading to customized premiums and improved risk management. This precision is particularly valuable in industries where risk assessment is complex or traditional methods are less effective.
Examples include fleet management, ride-sharing, and logistics, where the variability in driver behavior and vehicle usage can significantly impact risk.
Case Studies Illustrating Application
Several case studies highlight the successful implementation of black box insurance. For instance, a ride-sharing company saw a substantial reduction in claims after implementing a black box insurance program. The data collected allowed them to identify and address high-risk driving patterns, leading to safer operations and reduced insurance costs. Similarly, a fleet management company utilizing black box insurance data identified patterns of driver fatigue and poor maintenance practices, allowing for targeted interventions to improve safety and minimize vehicle downtime.
Application to New or Emerging Industries
Black box insurance holds immense potential for emerging industries with unique risk profiles. The sharing economy, particularly services like delivery platforms and ride-sharing, often presents challenges in accurately assessing risk. Black box insurance can effectively capture the nuances of these platforms, enabling more precise risk assessment and tailoring insurance products to meet specific needs. Similarly, in the realm of drone delivery or autonomous vehicle services, black box data can help insurers understand the factors contributing to risk, leading to more accurate premiums and innovative insurance products.
Impact on Risk Management Strategies
Black box insurance fundamentally alters risk management strategies by moving from a static, generalized approach to a dynamic, data-driven one. Insurers can proactively identify and address high-risk behaviors, leading to safer operations and potentially lower claims. This approach allows for more personalized risk assessment, leading to customized premiums and tailored coverage packages. This personalized approach can enhance customer satisfaction and potentially improve overall industry safety.
The impact on risk management is far-reaching, potentially affecting the way businesses operate and manage their liability.
Table of Use Cases
Industry | Specific Use Case | Data Collected | Benefits |
---|---|---|---|
Fleet Management | Tracking driver behavior, vehicle maintenance, and route optimization. | GPS data, engine performance metrics, driver behavior (speed, braking, acceleration), maintenance records. | Reduced accidents, improved fuel efficiency, lower insurance premiums, minimized vehicle downtime, improved operational efficiency. |
Ride-Sharing | Assessing driver behavior and vehicle safety in real-time. | GPS data, speed, acceleration, braking, location, and driver characteristics (e.g., age, experience). | Reduced accidents, lower insurance premiums, improved customer safety, optimized pricing models. |
Logistics | Monitoring delivery routes and driver behavior for package handling. | GPS data, vehicle usage, driver behavior (handling), delivery times, package conditions. | Improved delivery efficiency, reduced package damage, lower insurance premiums, enhanced customer satisfaction, optimized route planning. |
Drone Delivery | Monitoring flight patterns and environmental factors. | Flight path, altitude, speed, weather conditions, and other drone performance data. | Reduced accidents, improved safety standards, optimized delivery routes, tailored insurance coverage. |
Future Trends and Developments
Black box insurance, leveraging data analytics and telematics, is poised for significant evolution. Anticipated advancements in sensor technology, data processing, and AI algorithms will shape the future landscape of this dynamic insurance sector. These developments promise to refine risk assessment, personalize pricing, and enhance overall efficiency. However, challenges regarding data privacy, security, and potential biases in algorithms require careful consideration.
Anticipated Advancements in Black Box Insurance Technologies
Technological advancements are driving innovations in black box insurance. Improved sensor technology, capable of capturing more nuanced driving data, will lead to a more precise understanding of risk profiles. Real-time data analysis and sophisticated algorithms will enable insurers to assess risk dynamically, adjusting premiums based on current driving behavior. Furthermore, the integration of artificial intelligence (AI) and machine learning (ML) will facilitate more accurate risk prediction and personalized pricing models.
Emerging Challenges and Opportunities for Black Box Insurance
Black box insurance faces emerging challenges that must be addressed. Ensuring data privacy and security is paramount, given the sensitive nature of collected driving data. Addressing potential biases in algorithms, which might disproportionately affect certain demographics, is critical for maintaining fairness and equity. Conversely, opportunities abound. By implementing robust data security measures and promoting transparency, insurers can cultivate trust and attract a wider customer base.
Opportunities also exist in expanding coverage beyond vehicles, incorporating data from other connected devices to provide holistic risk assessments.
Predictions on How Black Box Insurance Will Evolve
Black box insurance is projected to become more sophisticated and personalized. Insurers will increasingly leverage real-time data and predictive analytics to tailor policies to individual driver behavior. Premium adjustments based on immediate driving patterns will become commonplace, reflecting the dynamic nature of risk. Expect a greater integration of black box insurance with other connected devices and services, such as smart homes and wearables, to provide a broader view of the customer’s risk profile.
The rise of AI-powered risk assessment tools will further refine the accuracy of risk predictions and enable the development of customized insurance solutions. For example, insurers could offer policies that reward safe driving behavior with discounts or offer specialized coverage packages tailored to specific driving needs.
Potential Regulatory Implications for Black Box Insurance
Regulatory frameworks will play a critical role in shaping the future of black box insurance. Stricter data privacy regulations, like GDPR and CCPA, will necessitate compliance measures from insurers to protect customer data. Further scrutiny of algorithmic fairness and transparency is anticipated, requiring insurers to demonstrate that their algorithms are free from bias. Insurance regulators will need to adapt existing regulations to address the unique challenges posed by data-driven risk assessment in black box insurance.
These regulations will likely include requirements for data security, transparency in pricing models, and mechanisms for addressing potential discrimination.
Potential Future Developments in Black Box Insurance and Related Technologies
A number of potential future developments are anticipated in the field of black box insurance and related technologies. These include:
- Development of advanced sensor technology: Sensors capable of capturing a wider range of driving data, including subtle nuances of driving behavior and environmental factors, will provide a more comprehensive risk assessment.
- Refinement of data analysis techniques: More sophisticated data analysis techniques, utilizing machine learning and AI, will enhance the accuracy of risk prediction and personalization of insurance products.
- Integration with other connected devices: Expanding the scope of data collection beyond vehicles to include data from other connected devices, such as smart homes and wearables, will provide a holistic view of risk.
- Expansion of coverage to new sectors: The use of black box technology may expand beyond automobiles to other sectors, such as home insurance, where real-time data analysis can identify and mitigate risks.
- Development of personalized insurance products: AI-powered risk assessment will allow insurers to develop customized insurance policies that cater to the specific needs and risk profiles of individual drivers.
Illustrative Scenarios
Black box insurance, leveraging telematics data, presents a unique set of scenarios that illustrate both its potential and limitations. These examples highlight how the data-driven approach affects policyholders, insurers, and the overall insurance landscape. Understanding these situations is crucial for evaluating the efficacy and ethical implications of this emerging insurance model.
Hypothetical Scenario of Black Box Insurance Usage
A young driver, Sarah, recently obtained her driver’s license and is eager to secure car insurance. She selects a black box insurance policy. The policy’s terms are linked to her driving habits, including speed, braking, and acceleration. The device records her driving patterns, and the insurance company analyses the data. If Sarah consistently demonstrates safe driving practices, her premiums are reduced over time, rewarding responsible behavior.
Conversely, if her driving patterns indicate risky behaviors, the insurance company may adjust her premium upward. This dynamic adjustment reflects the real-time assessment of her risk profile.
Scenario Illustrating a Risk Not Covered by Black Box Insurance
A black box insurance policy may not cover incidents where external factors significantly influence the insured vehicle’s status. Consider a scenario where a sudden and unexpected hail storm damages a car. The black box may record the car’s condition prior to the storm, but it cannot account for the sudden and unforeseen weather event. The policy’s coverage might not extend to such natural disasters, which fall outside the parameters of the data collected by the device.
This highlights the limitations of relying solely on driving behavior data for comprehensive coverage.
Case Study: Impact on Claims
A policyholder, Mark, experienced a car accident. The black box data, meticulously collected and analyzed, provided crucial evidence. The data showed Mark was not speeding, and the other driver was at fault. The insurance company, using the data, promptly approved Mark’s claim and compensated him for damages. This exemplifies how black box data can objectively assess liability, reducing disputes and expediting the claims process.
Such evidence-based claims processing can lead to greater efficiency and fairness in insurance settlements.
Dispute Arising from Data Analysis
A policyholder, Emily, experienced a claim dispute. The black box data indicated a sudden and unexpected braking event. However, Emily contended that the braking was necessary to avoid an accident caused by a reckless driver. The insurance company analyzed the data, but the interpretation remained ambiguous. The black box data was insufficient to definitively prove the nature of the event.
This highlights the need for clear policy terms and human oversight to address potential discrepancies in data interpretation and ensure fairness.
Yo, like, black box insurance is this thing where they track your driving habits, right? It’s all about your driving style, how much you drive, and stuff. But, if you’re looking for a sweet Section 8 house for sale, check this out. It’s lowkey important to know your insurance options before you buy, so you can keep the payments smooth, you know?
So yeah, black box insurance is all about that.
Influence of Data Collection on Future Policies
Extensive data collection in black box insurance can influence future policy design and pricing models. If the data reveals a correlation between certain driving patterns and accidents in specific geographic locations, insurers may adjust premiums accordingly for drivers in those areas. For example, if data indicates a high rate of accidents at a particular intersection, insurers might introduce preventative measures or adjust policy pricing for drivers using that route.
This proactive approach reflects the dynamic nature of risk assessment and policy development.
Final Thoughts

In conclusion, black box insurance represents a significant shift in the insurance landscape. Its potential to offer personalized and potentially more affordable policies is undeniable. However, concerns regarding transparency, accountability, and data security require careful consideration. As the technology matures and regulations adapt, black box insurance is poised to reshape risk management strategies, potentially transforming how we perceive and mitigate risks in various sectors.
FAQ Corner
What are the common coverage types in black box insurance?
Common coverage types might include auto insurance, property insurance, or even specific types of commercial insurance, depending on the data collected and the risks being assessed. The precise types will vary based on the insurer and the intended application.
How does black box insurance differ from traditional insurance?
Traditional insurance relies heavily on pre-defined risk factors and historical data. Black box insurance uses a more dynamic approach, analyzing real-time data to assess risk and adjust premiums accordingly. This means that your behavior, rather than just your demographics, significantly impacts your premiums.
What are the potential risks of using black box insurance?
Potential risks include concerns about data security, potential for bias in algorithms, and the lack of transparency in how decisions are made. The lack of clear explanation for premium adjustments can create distrust and potentially lead to unfair outcomes.
What are the data sources used in black box insurance?
Data sources could include GPS location data, driving behavior, and even environmental conditions. The exact data used will depend on the specific type of insurance and the goals of the insurer.